Contributors: Shawani Shome, Karthik Namburu, Rosario Muthu Rajan Vincent Xavier
Introduction:
In the fast-paced world of scientific research, the transformation of raw data from instruments into actionable insights is a crucial process. This journey involves several critical stages:
- Enabling the Lab Scientist
- Structured Data Capture
- Data Foundation
- Scientific Insights
Each stage plays a vital role in managing diverse types of data across both large and small molecule research, as well as handling complex modalities within preclinical studies. Understanding and optimizing these stages is essential as the demand for quickly and efficiently generating insights from experimental data continues to rise.
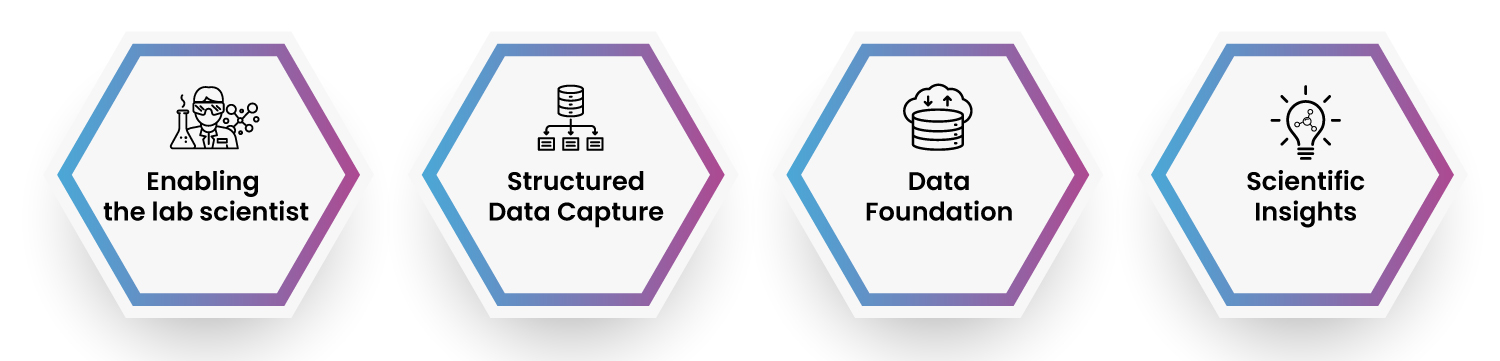
Lab Enablement
In today’s research environment, lab scientists deal with various types of data from different experiments, using a range of instruments. Whether it’s observations or sample/equipment information, tracking and managing this data is essential. Lab enablement is the first step in this journey, involving the assembly and optimization of laboratory instruments. Ensuring that labs are equipped with the latest tools and technologies, from advanced chromatography systems to next-generation sequencing platforms, sets a strong foundation for the subsequent stages of data processing.
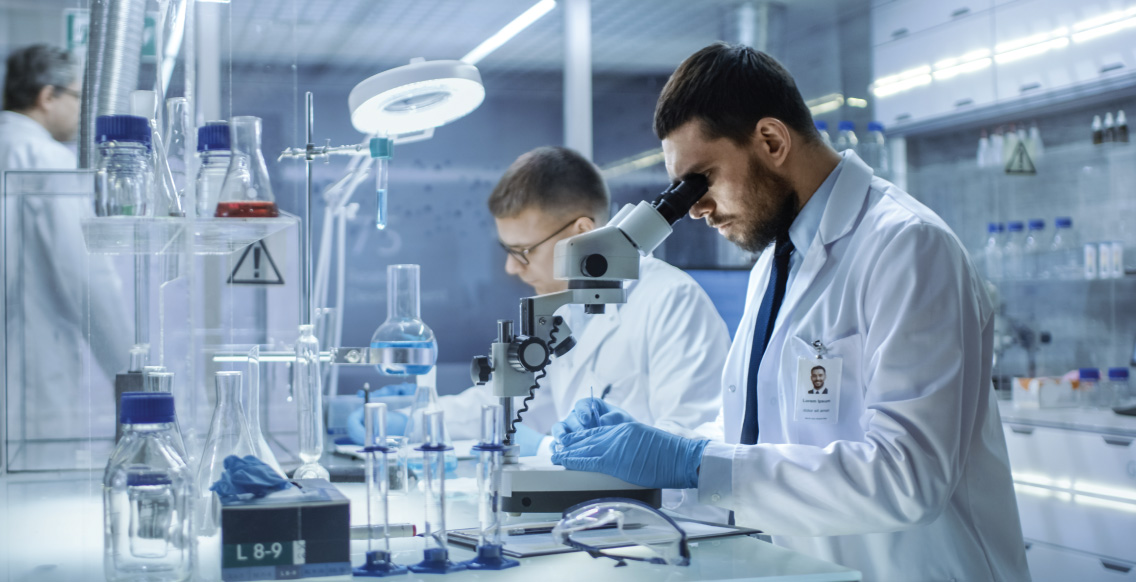
Data Capture and Automation
Once data is generated on a large scale, it’s crucial to capture, store, and manage it efficiently. This is where data capture and automation come into play. Tools and technologies involved at this stage ensure that data is collected accurately and effectively. The quality of lab instruments directly influences the accuracy of data capture. Moreover, the automated transfer of this data from instruments to databases minimizes errors and enhances efficiency.
Recently, Electronic Lab Notebooks (ELNs) and Laboratory Information Management Systems (LIMS) have emerged as key enablers of lab digitalization. These systems help document and manage experimental data, significantly reducing human error and data loss. Additionally, they free up valuable time for scientists, allowing them to focus on research rather than manual data transcription.
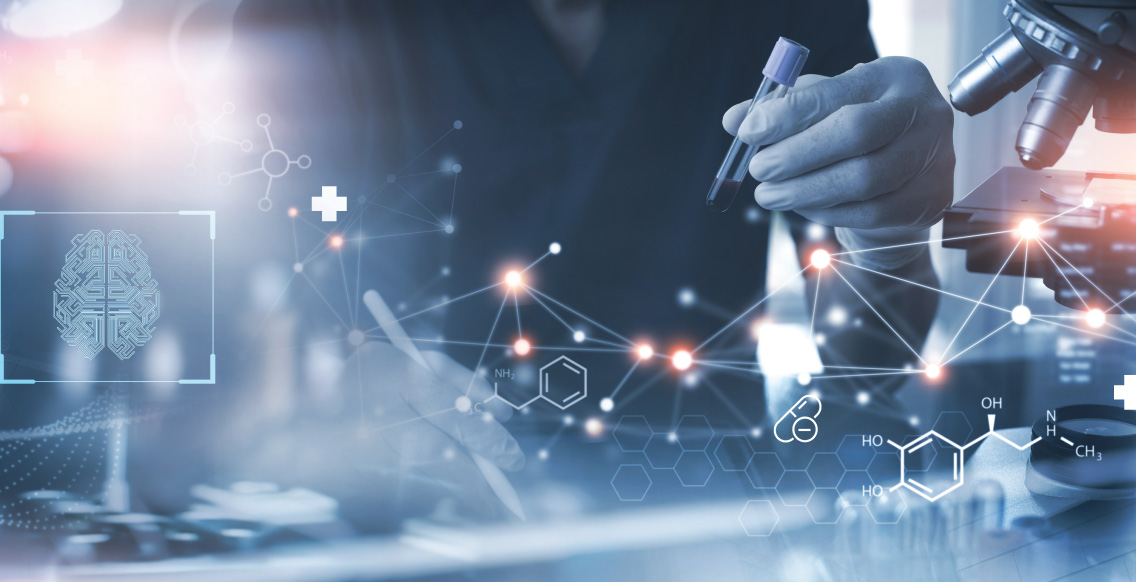
Data Foundation
Once scientific data is registered, it’s essential to integrate and structure it to create a robust data foundation. A centralized repository, often referred to as a “Data Lake,” is used to store vast amounts of raw data. Platforms like Snowflake help store and manage this data, which, after processing, is stored in a data warehouse for future retrieval and analysis. Standardizing this data is crucial to maintaining consistency and ensuring interoperability across the organization.
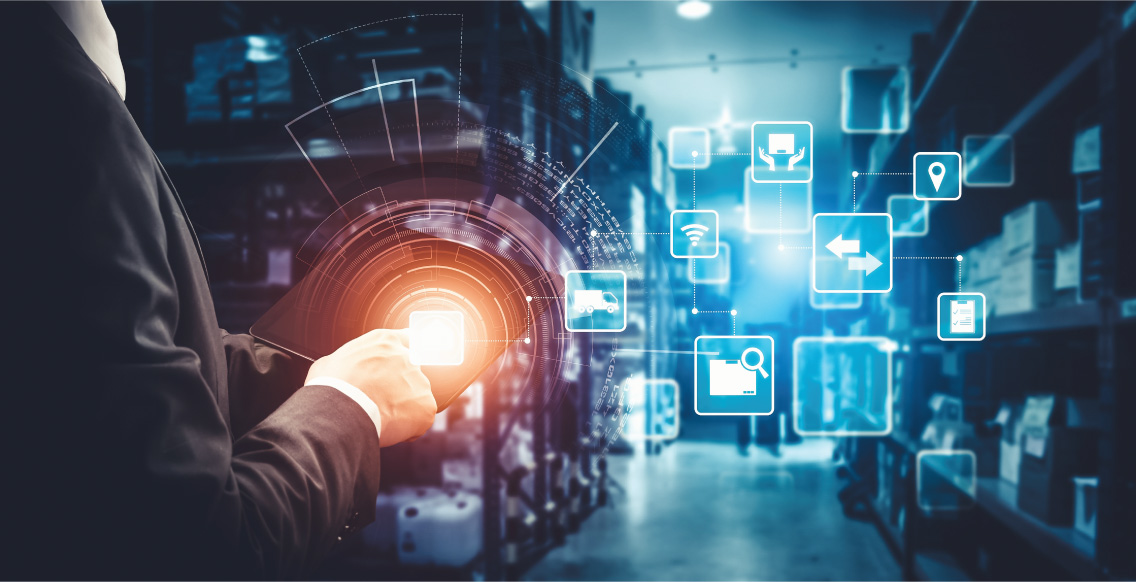
Scientific Insights
The final stage is analyzing the processed data to derive meaningful scientific insights. This stage is supported by dedicated tools and platforms that allow researchers to search and explore data from large repositories. With advancements in technology, a variety of software applications and dashboards have been developed to facilitate data analysis and visualization, making it easier to interpret results. Sometimes, coding, scripting, and customization are required to present raw data in a visually understandable format, reducing manual intervention.
This processed data is then provided to lab scientists, aiding them in strategizing their studies and designing future experiments more effectively. The entire process is underpinned by a range of specialized tools, including LIMS, ELNs, data capture tools, data processing software, and data visualization platforms. These tools are designed to handle various data types, such as chemistry data, disease biology data, imaging data, omics data, and assay results.
A solid infrastructure—comprising on-premises and cloud-based solutions, high-performance computing systems, and robust databases—accelerates the research process significantly. Creating and maintaining such systems require multidisciplinary skill sets, including identifying relevant data sets, understanding requirements, and applying appropriate analysis methods to generate insights using emerging technologies like Artificial Intelligence, Machine Learning, and Data Science.
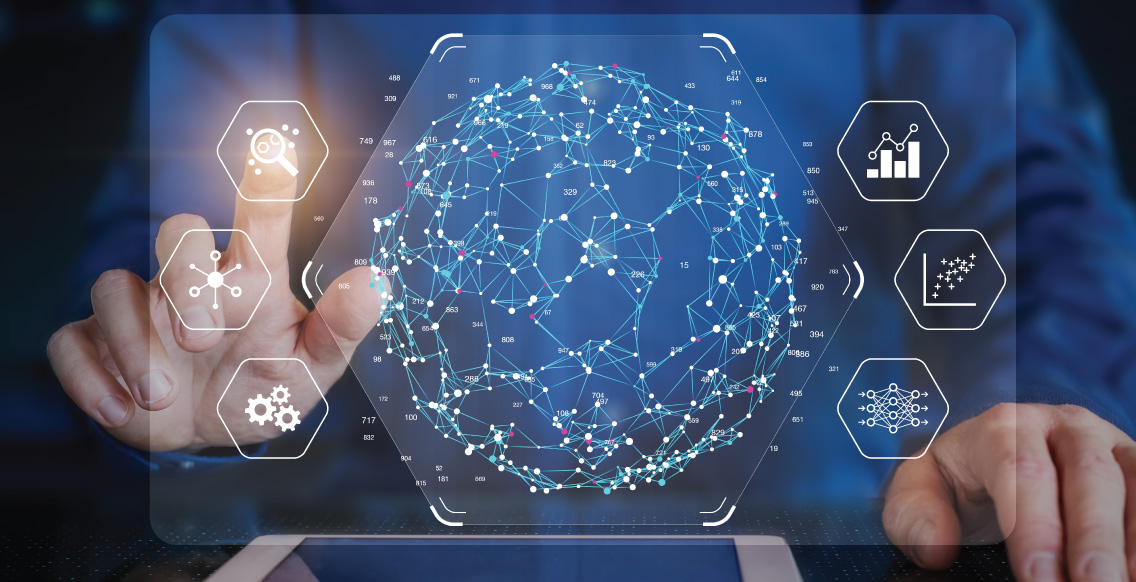
Conclusion
The journey of transforming raw data from laboratory instruments into actionable scientific insights is complex yet highly rewarding. By following the stages outlined above and leveraging the right tools and skill sets, researchers can unlock the full potential of scientific data. This process not only deepens our understanding of scientific domains but also drives innovation and discovery across various fields.
Excelra, with its state-of-the-art expertise and experienced team, is here to support you on this journey from instruments to insights. Whether you’re at the beginning of the process or anywhere in between, Excelra has the knowledge and technical capabilities to help you succeed.
Contact us today to discuss how our ELN & LIMS Managed Services can empower your lab to reach its full potential.