The client, a Biotech company based out of Europe, had a large molecule asset that was under development for blood cancer. They were interested in expanding the therapeutic potential of the molecule to solid tumours to augment the existing portfolio.
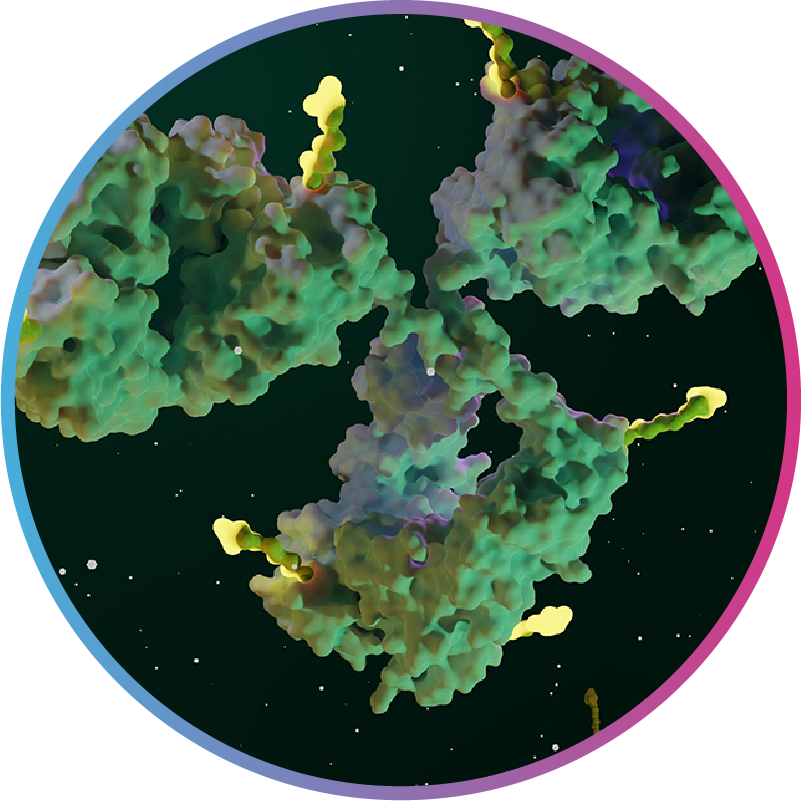
Client’s requirement
The focus was on leveraging public gene expression data-sets from cancer patients treated with a drug candidate with similar mechanism of action. Machine-learning based predictive models were built using information on drug responsiveness and disease gene signatures.
Our approach
Predictive models were built using an iterative approach wherein patient-level and disease-level gene expression profiles were used as input data. Clustering of cancer indications was done to prioritize indications which were potentially sensitive to the treatment. Biological rationale was built was each of the prioritized indications by converging the drug mechanism of action with disease pathophysiology.