Master data is considered as core data for a company upon which the company relies for multiple operations. The data helps in extracting meaningful insights about the process carried out in the firm. These data can serve as a single source of truth for various systems like Electronic Laboratory Notebooks (ELN) and Laboratory Information Management Systems (LIMS) in the field of research. However, these systems’ effectiveness relies on the data’s quality and integrity.
Master Data Preparation involves multiple steps of process that include data collection, data harmonization, data mapping, and integrating data from various sources to create data with quality to serve as a single source of truth. This process is essential in the research and pharma industry as it ensures that all data is accurate and up to date, which is crucial for making informed decisions. This blog post can provide an overview of the important aspects of data collection steps, highlighting its significance in efficiency and compliance with scientific workflows.
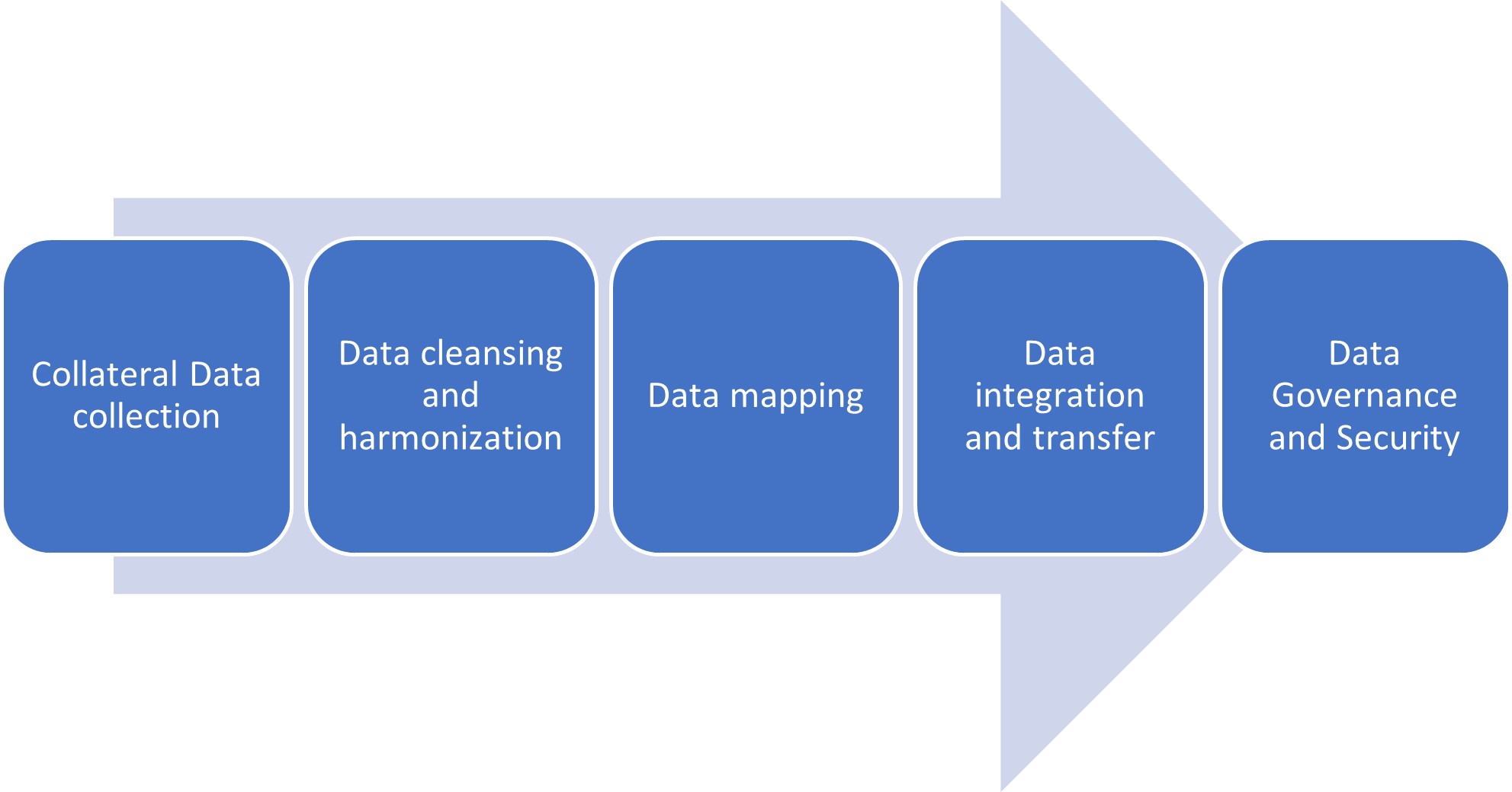
1. Collateral Data collection
This journey begins with the identification and gathering of relevant collateral entities. Data is sourced from external databases or from documents like COA, Test data sets, Standard test procedures, and Specifications and is collated to form a comprehensive dataset. The data collected can include product information, customer data, supplier details, and other regulatory compliance data.
Various methods are used to collect this data, such as workshops, data mining, and manual data entry. It is vital to ensure that the data collected is accurate, complete, and up to date to avoid future incompetencies. The collected data are then studied to understand their whole purpose before we begin the harmonization process.
2. Data Cleansing and Standardization
This phase involves understanding the collected data and removing and re-organizing them to rectify any identified issues. Standardization and cleansing processes ensure uniformity, accuracy, and completeness. This process involves the removal of duplicate data, correcting the existing errors, and adding the missing information to the data.
Various methods are used to ensure data quality during the harmonization process. This may include
- Using of automation tools to detect and correct errors,
- Performing manual review to verify data accuracy, and
- Implementing data validation rules.
These steps ensure the accuracy and consistency of the data.
3. Data Mapping
This phase starts with the harmonized data taken from the previous step and mapping with the new dataset of the identified system. The data mapping is done considering the functionality of the data is preserved during this process. This data is then modified and restructured to reflect the structure of data in ELN/LIMS. This might involve a manual process or an automated process based on the data followed in lab workflow. It is a crucial step as they lay out the backbone structure for data to be imported into the system.
4. Data Integration and transfer
The harmonized data is then manually transferred into the targeted system or through integration. Considering the data complexity and volume, Integration ensures the successful data transfer without compromising data quality or quantity. This also automates the whole process of transferring data into the COTS system. The data format to be transferred should align with the data format of the COTS system before transfer.
5. Data Governance and Security
Data governance is vital in accuracy, reliability, accessibility, and security. It is essential to have strict policies and procedures laid out to ensure data privacy and security. Implementing adequate security measures, access controls and permissions, regular data backups, and disaster recovery plans ensures data safety. It is necessary to adhere to the company’s governance policies and other regulatory requirements, such as HIPAA, for patient data. By implementing strong data governance and security measures, the pharma industry can protect its valuable data assets and maintain the trust of its stakeholders. This systematic approach to managing data helps organizations derive value from them and make informed decisions.
To conclude, adoption is one of the crucial aspects of Darwin’s evolution theory. In this fast-paced world, the research labs and pharma industries recognize the importance of adapting new technologies without compromising their existing core data. Effectively managed Master data can be incorporated and transferred into new technologies to lead the way for future medicines.
That’s why you need more than just data. That’s why you need Excelra. Where data means more.