Expertly curated data and advanced analytics have had a huge impact on drug repurposing – the process of finding new uses for existing treatments. The importance of drug repurposing cannot be overstated, and significant advances in patient outcomes are achieved thanks to this branch of pharmaceutical science
Traditionally, there have been two common approaches to drug repurposing. The first is drug- or target-centric repurposing, which analyses the drug or target to identify alternative applications. The second is disease-centric repurposing, which considers the mechanisms of disease and attempts to identify existing drugs that may impact them. A third approach has gained momentum in recent years, which seeks to find new combinations of drugs to broaden the variety of applications.
Whichever of these approaches is taken, the use of precisely selected and effectively analyzed data has been fundamental to the rapid growth in the number of repurposed drugs tested in the clinic.
Drug centric
Focus on identifying alternative diseases taking drugs as input
Target centric
Focus on identifying alternative diseases taking target as input
Disease centric
Focus on identifying alternative drugs/target taking disease as input
Machine learning and data-driven analytics
Advanced machine learning and data-driven analytics are having a profound impact on drug repurposing. The breadth and depth of the data produced by biological experiments has increased dramatically, allowing modern analytical tools to interrogate and interpret it in many different ways.
Applications of machine learning in repurposing
Drug-target and drug-disease interaction prediction
Establishing drug-target-disease relationships forms the cornerstone of all repurposing programs. With a wide range of data available to build these connections – including OMICs and biochemical assay endpoints – computational biologists have developed innovative methodologies to identify and accurately predict drug-target and drug-disease associations. These methods complement experimentally mapped drug-target and drug-disease interaction networks and are a valuable addition to repurposing programs1.
- Drug target interaction prediction
Drug–target interactions using graph embedding, graph mining, and similarity-based techniques (DTiGEMS+) is a computational method that builds heterogeneous networks in the form of drug-prediction graphs. It does so by enriching the network maps of known drug-target interactions with data from drug-drug similarity and target-target similarity2. A similar approach is DGraphDTA, which uses neural networks to map the structural information of proteins and drugs in order to predict their activity3. - Drug-disease interaction prediction
Similarity network fusion (SNF), and neural network (NN) deep learning models accurately identify drug-disease associations by exploiting data for drug-similarity, disease-similarity and known drug-disease interactions4. A dense convolutional neural network (DCNN) is another example of a deep learning model which combines data on the structural similarity of drugs and the semantic similarity of diseases. It captures the key characteristics of drugs and diseases and applies adaptive optimization of those features to allow better predictions of drug-disease associations5. - Drug mechanism of action elucidation
A common task of R&D teams in pharma companies is to utilize the gene signatures of drugs and diseases to assess their mechanism of action (MoA). Detecting mechanism of action by network dysregulation (DeMAND) is one example of the network-based approach to assessing MoA. Another is a genetic profile-activity relationship (GPAR) tool, which, a tool which utilizes gene expression data for modeling and predicting mechanisms of action via deep learning7. - Drug combination prediction
The application of new combinations of drugs to improve efficacy and reduce toxicity during therapy is a key objective of drug developers. Experimental screening for identifying such suitable drug combinations can, however, be expensive and time-consuming. Fortunately, network-based computational approaches such as SynGeNet, and ML-based approaches such as DeepSynergy, have led to a dramatic acceleration in the prediction process9-11. - Drug-disease interaction prediction
Identification of drug response biomarkers in oncology for patient selection and stratification is of huge interest to drug developers as it has a direct impact on the success of clinical trials. A traditional roadblock was the data: correlating drug response biomarkers with outcomes requires sufficient sample size, effective computational tools and recognition of the context, and where there was insufficient data, the machine learning options were limited. To overcome these obstacles, several context-driven univariate and multivariate analysis methodologies and deep learning methods are published to classify responders vs non responders to a therapy8.
Advanced data analytics and machine learning applications also include cell and tissue-based response predictions and hit identification. It is a rapidly evolving field, built on the enthusiasm for its promise of increased accuracy, reduced time, and ability to illuminate novel associations which might otherwise have remained obscured.
Excelra’s role in accelerated drug repurposing
Excelra leverages our custom-built Global Repurposing Integrated Platform (GRIP) algorithms to mine public and proprietary databases to curate high-quality data for use in advanced analytics and predictive ML model building. Our experts work at the intersection of science and technology, and are uniquely adept at bridging the gap between those two fields.
Excelra is one of the most enthusiastic proponents of the data revolution, and is a passionate advocate of utilizing advancing technologies in data analytics for drug repurposing programs. Data mining, structuring, transformation and predictive model building forms a core part of our expertise.
Combining subject matter expertise with innovate technological solutions, we provide deep biological insights and powerful data outputs to provide exciting, value-driven recommendations to our clients.
Case study
Objective
Identification of alternate indications for a clinical compound.
Challenge
A pharmaceutical company from the west coast of the USA intended to repurpose their clinical candidate to identify alternative indications.
Solution
Using data extracted by Excelra’s GRIP platform, alternate indications were identified for the company’s clinical compound. The pharma was also able to predict drug-disease associations by processing the data with advanced machine learning models (Fig 1).
Excelra’s GRIP platform incorporated in silico analyses with literature mining, clinical trials, structural similarity, and interactome. Various machine learning approaches were employed to identify potential drug-disease pairs, including drug-drug similarity, disease-disease similarity, and target mechanism of action-related pathway features. These approaches helped to predict several relevant drug-disease associations beyond those reported in the public domain for the scrutiny of subject matter experts.
The process returned recommendations for five drug-disease associations, supported by scientific rationale. The associations included two rare diseases, an autoimmune disease, a gastrointestinal disease, and a cardiovascular disease.
Value created
Excelra’s curated data was used by advanced ML models to assess alternative drug-disease pairs. The astute application of data led to the identification of novel indications for the customer’s clinical compound from diverse therapeutic areas and created significant additional value within the program.
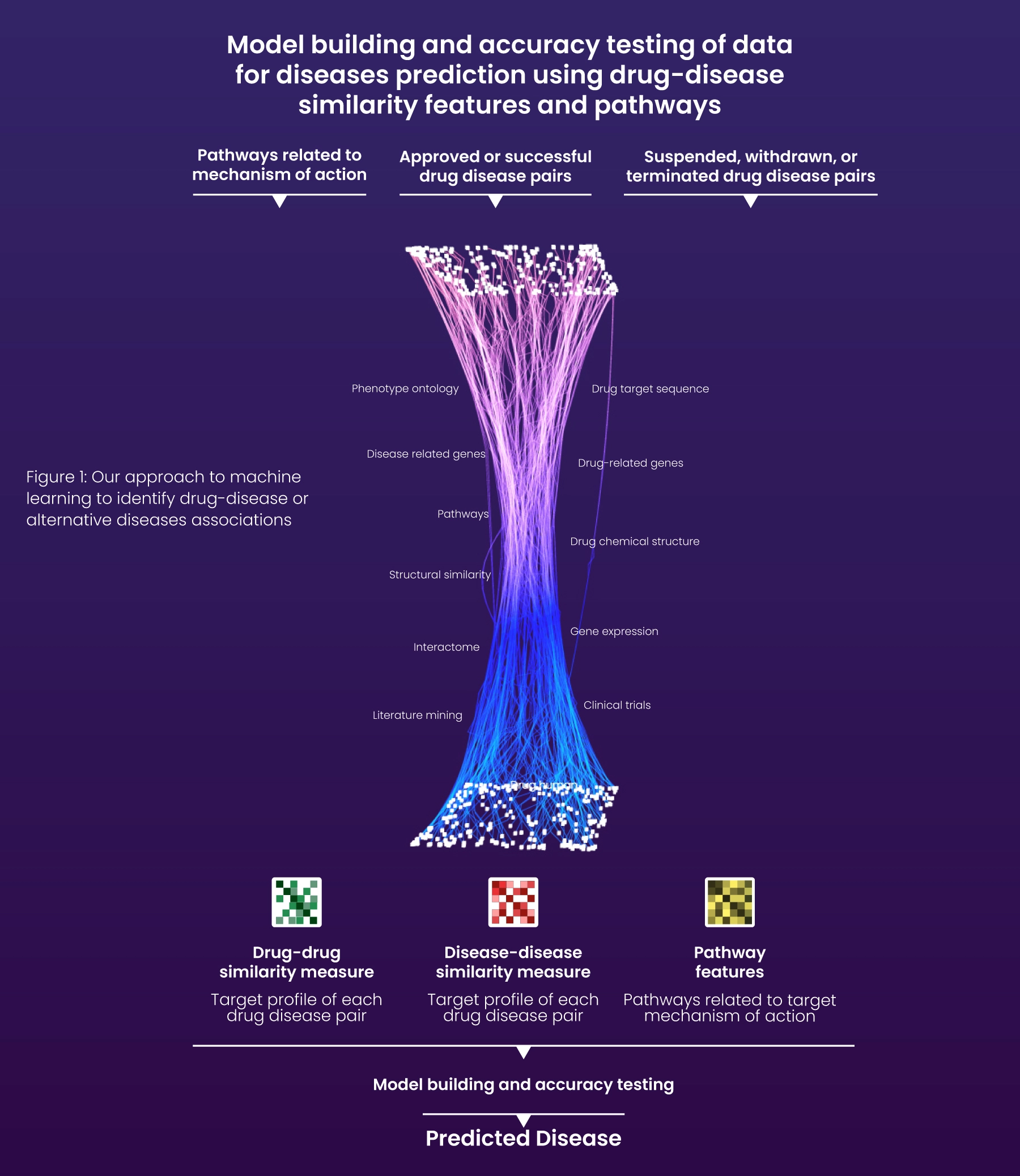
Fig: Our approach to machine learning to identify drug-disease or alternative indications associations
Summary
Drug repurposing has been revolutionized by the availability of carefully curated data and the application of that data in advanced analytics and machine learning models. Exploiting data to its fullest potential has led to the identification of novel associations and dramatically accelerated the return of accurate interpretations.
References
- Tanoli, , Vähä-Koskela, M., & Aittokallio, T. (2021). Artificial intelligence, machine learning, and drug repurposing in cancer. Expert opinion on drug discovery, 16(9), 977–989. https://doi.org/10.1080/17460441.2021.1883585
- Thafar, A., Olayan, R. S., Ashoor, H., Albaradei, S., Bajic, V. B., Gao, X., Gojobori, T., & Essack, M. (2020). DTiGEMS+: drug-target interaction prediction using graph embedding, graph mining, and similarity-based techniques. Journal of cheminformatics, 12(1), 44. https://doi.org/10.1186/s13321-020-00447-2
- Jiang, , Li, Z., Zhang, S., Wang, S., Wang, X., Yuan, Q., & Wei, Z. (2020). Drug-target affinity prediction using graph neural network and contact maps. RSC advances, 10(35), 20701–20712. https://doi.org/10.1039/d0ra02297g
- Jarada, N., Rokne, J. G., & Alhajj, R. (2021). SNF-NN: computational method to predict drug-disease interactions using similarity network fusion and neural networks. BMC bioinformatics, 22(1), 28. https://doi.org/10.1186/s12859-020-03950-3
- Wang, , Zhao, S., Zhao, J., & Feng, Z. (2021). A model for predicting drug-disease associations based on dense convolutional attention network. Mathematical biosciences and engineering : MBE, 18(6), 7419–7439. https://doi.org/10.3934/mbe.2021367
- Woo, J. H., Shimoni, Y., Yang, W. S., Subramaniam, P., Iyer, A., Nicoletti, P., RodrÃguez MartÃnez, M., López, G., Mattioli, M., Realubit, R., Karan, C., Stockwell, R., Bansal, M., & Califano, A. (2015). Elucidating Compound Mechanism of Action by Network Perturbation Analysis. Cell, 162(2), 441–451. https://doi.org/10.1016/j.cell.2015.05.056
- Gao, , Han, L., Luo, D., Liu, G., Xiao, Z., Shan, G., Zhang, Y., & Zhou, W. (2021). Modeling drug mechanism of action with large scale gene-expression profiles using GPAR, an artificial intelligence platform. BMC bioinformatics, 22(1), 17. https://doi.org/10.1186/s12859-020-03915-6
- Adam, , Rampášek, L., Safikhani, Z., Smirnov, P., Haibe-Kains, B., & Goldenberg, A. (2020). Machine learning approaches to drug response prediction: challenges and recent progress. NPJ precision oncology, 4, 19. https://doi.org/10.1038/s41698-020-0122-1
- Güvenç Paltun, , Kaski, S., & Mamitsuka, H. (2021). Machine learning approaches for drug combination therapies. Briefings in bioinformatics, 22(6), bbab293. https://doi.org/10.1093/bib/bbab293
- Bai, L. Y., Dai, H., Xu, Q., Junaid, M., Peng, S. L., Zhu, X., Xiong, Y., & Wei, D. Q. (2018). Prediction of Effective Drug Combinations by an Improved Naïve Bayesian International journal of molecular sciences, 19(2), 467. https://doi.org/10.3390/ijms19020467
- Wang, , Li, H., & Guan, Y. (2020). Machine Learning for Cancer Drug Combination. Clinical pharmacology and therapeutics, 107(4), 749–752. https://doi.org/10.1002/cpt.1773