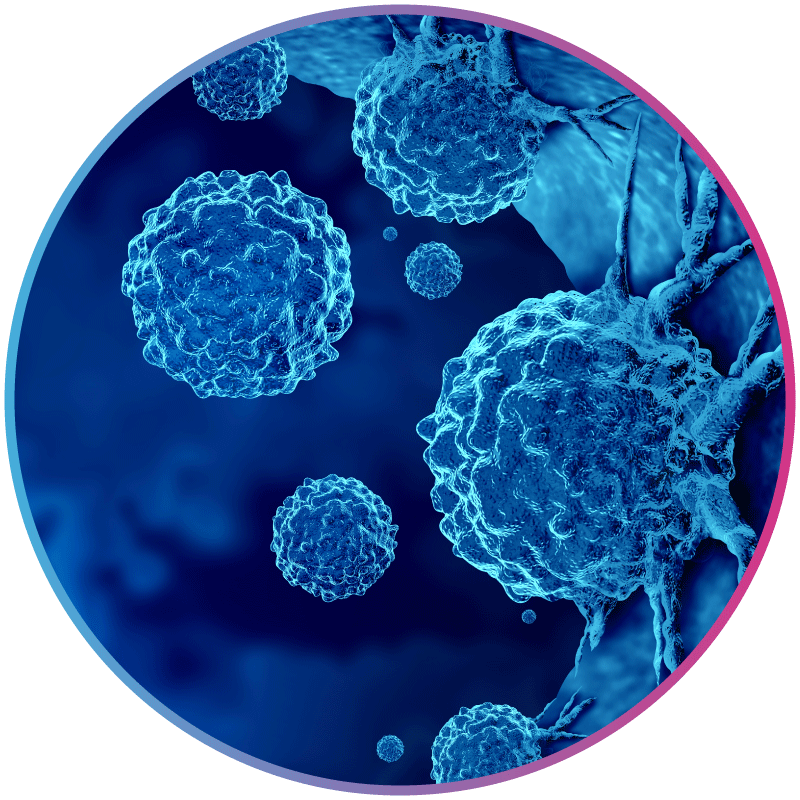
Our client’s requirement
A California-based pharmaceutical company specializing in immunotherapy drugs faced a significant challenge: accurately identifying patients most likely to benefit from their treatment. While immunotherapy holds promise for various cancers, the varying responses among patients led to suboptimal treatment outcomes.
The Challenge:
- Inconsistent Treatment Response:Â The company observed significant variability in patient responses to their immunotherapy treatment.
- Suboptimal Patient Selection:Â Traditional patient selection methods based on broad clinical factors were insufficient in identifying ideal candidates.
- Missed Opportunities and Adverse Effects:Â This resulted in missed opportunities for patients who could benefit from the treatment and potential exposure to adverse effects for those who would not.
Client Objective:
To address these challenges, the company aimed to develop a robust system capable of accurately classifying patients into two distinct groups:
- Responders:Â Patients highly likely to experience a positive treatment response.
- Non-responders:Â Patients with a low probability of treatment response and potential risk of adverse events.
By achieving this classification, the client sought to:
- Improve treatment efficacy:Â Maximize the number of patients who benefit from the immunotherapy.
- Enhance patient safety:Â Minimize the risk of adverse events by avoiding unnecessary treatment in non-responders.
- Optimize clinical trials:Â Streamline trial processes by focusing on patient populations most likely to benefit from the treatment.
Our approach
Excelra partnered with a client to develop an AI-powered approach for identifying patients most likely to respond positively to their immunotherapy treatment. This collaboration addressed a critical challenge in the field – ensuring the right patients receive the right treatment.
- To achieve this, Excelra built a comprehensive patient profile by integrating data from various sources like electronic health records, genomics, and clinical trials.
- They then employed sophisticated techniques to identify the most relevant data points for accurate prediction. Machine learning models were developed and evaluated, with XGBoost demonstrating the best performance.
- Importantly, Excelra went beyond just achieving high accuracy. They used advanced methods to understand how the model arrived at its classifications, providing valuable insights into the factors influencing patient response.
- The results were impressive: the XGBoost model achieved 89% accuracy in differentiating responders from non-responders. This translates to potentially improved treatment outcomes and reduced side effects.
- By leveraging AI, Excelra has helped pave the way for a more personalized approach to immunotherapy, ultimately leading to better patient care.